The accuracy of the predictions will depend on the data used when constructing a predictive model. If you use insufficient or inaccurate data, your model will be incapable of making accurate predictions. This will take you in the wrong direction. To prevent this, you must comprehend the distinction between testing and training data in machine learning or get guidance from professional AI model training services. Let us learn about it in detail in this article.
Testing Data For AI Models
Every time a machine learning model has been constructed with training data, new data is needed to test it. This information is called testing data, which one can use to:- Assess the performance and development of your algorithms’ training
- Modify or optimise it for better results
- Display the actual data set
- Be sufficiently large to generate useful predictions
All About The Training Data
Machine learning employs algorithms to acquire knowledge from datasets. They identify patterns, make decisions, gain a good understanding, and evaluate the decisions. Machine learning divides datasets into two subsets.- The first subset is referred to as the training data. The actual dataset is given to the ML model to identify and develop patterns. In this manner, it will train models.
- The second subset is referred to as the assessment data.
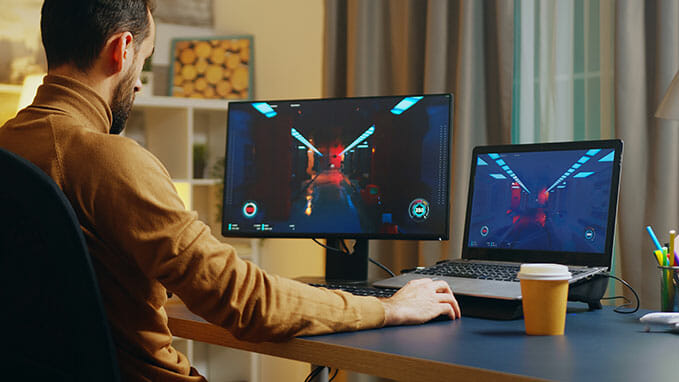